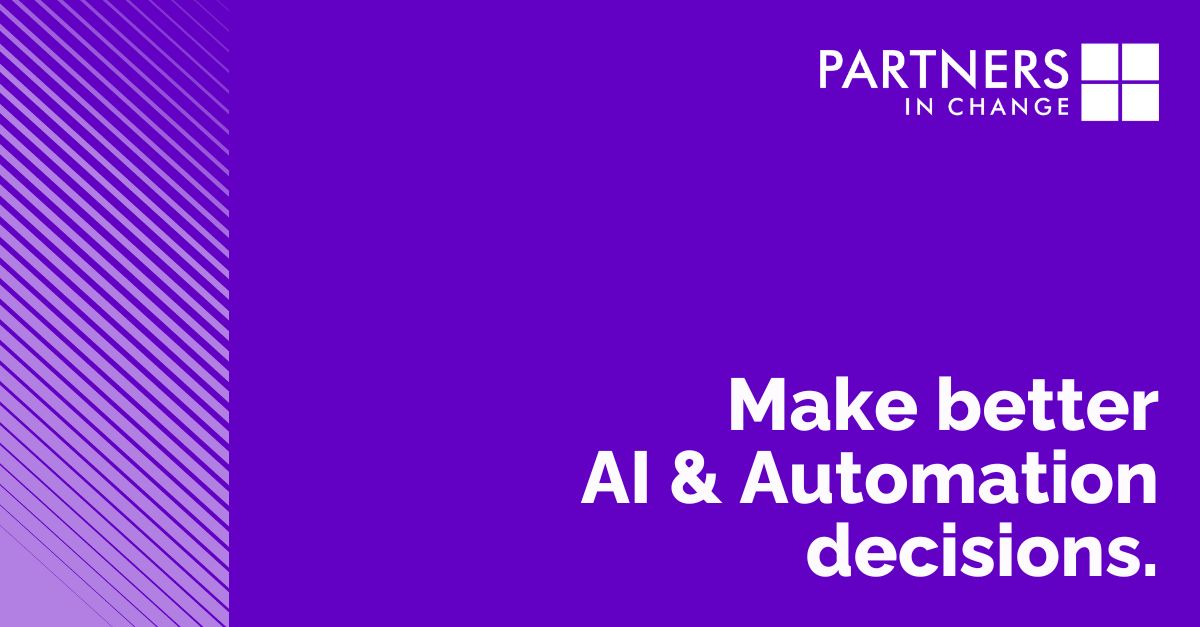
Organisations know that they risk being left behind if they fail to embrace AI and automation. But decisions are difficult to make and far-reaching in impact.
In a marketplace dominated by hype and obfuscation, identifying the best path forward is challenging. Factor in the bewildering pace of AI innovation and it can be tempting to defer decisions and await a point of clarity that is unlikely to ever come.
To help make such decisions easier we recently put together a panel of experts with experience working with AI and automation across a wide range of organisational applications and use-cases.
Richard McKenzie-Small, Mark Baginski and Martin Cropper have, between them, been involved with many automation activities covering multiple aspects of front, mid and back office.
We asked them to identify key considerations based on their personal experience; lessons learnt ‘in-field’ that would help organisations thinking about investing in such technology make better decisions.
Here are their ten key points to consider ahead of pushing ahead with investment:
- Really understand your customer and/or colleague
Success, whether measured by uptake/adoption, increased efficiency or quality or reduced waste or cost, depends on how well a new automated solution is designed. It is very clear that the best ones are based on a thorough understanding of the customer/colleague’s needs, expectations and behaviours. This will also drive choices made when selecting the target use cases.
- Start simple, start small and augment
All three panel members have had positive experience of projects where the AI/automation use-case could be delivered with existing data or interfaces and wasn’t going to challenge any complex areas of the enterprise rules, regulations or technology. Such ‘simple’ use cases that will enhance customer or colleague convenience, while delivering rapid and visible benefits, are a great place to start. Once these have been proven and lessons learned, the next tranche of use cases should be easier to get approved.
- Pick the ‘knottiest problems’ and target areas that have a real and pressing need
A supplemental point to 2., which may be an alternative strategy if you are confident with the solution, was that modern AI and automation projects can be used to solve problems that have exceeded the capabilities of previous generations of technology. For example, if there is a complex, dynamic process with multiple data sources, it may be that a tool can be trained (and learn) quickly and prove itself in a pilot before being rolled-out more widely. Done right, this approach can demonstrate real pace, both in implementation and delivery of benefits.
- Not every automation requires AI
In the panel’s experience, many smart workflow/automation projects are packaged with AI tools, almost by default.
This is likely to increase the cost, increase the time to launch and add a level of complexity that isn’t necessary justified. Often, a significant proportion of the business benefit can be delivered without AI/ML, perhaps with more advanced later phases to follow, once initial lessons have been learned and the business case is gaining momentum.
- Think very carefully before trying to replace people – augment not replace
There have been several news-worthy ‘fails’ where a complex and risky technology such as GenAI has been let loose on customers before it was ready or properly tested/controlled. Our advice is to keep a human-in-the-loop for customer-facing automations until they have demonstrated reliability, quality and performance. This may be a colleague monitoring real-time performance or it may be ‘escape routes’ should something go awry. Internal AI-powered apps should also be carefully tested and proven – Martin shared his experiences of clients trying to replace human interviewers with automatons, rather than supporting human interviewers with better information and prompts. It didn’t go well.
- Really understand your data and business rules (and regulations)
However good the AI/automation tool, there are two things that it won’t work without: (i) quality data and (ii) an understanding of your business rules. So early tasks in any project will be to map out the data sources and any gaps and document the decision points, rules and controls that govern your processes and will enable you to test the tool and assess its quality and performance.
- Don’t (just) listen to vendor salespeople
While this is a generalisation (there are some genuinely good products out there), the sales pitch will typically overhype the pace and benefits, while underplaying the cost, complexity and risk. As experienced implementers of AI- and non-AI powered solutions, our panel had several pieces of advice, for example:
- Do your research – talk to people who have done similar things, even if they are in different sectors
- Think about how you are going to deliver, what skills you will need around you
- Consider what changes you will need to make and how your customers and colleagues will react
- Truly tailored and personalised solutions are real and can add value
For a long time, we have been warned away from tailored technology solutions with difficult upgrades, compatibility clashes, divergence from best practice cited as issues. However, a system capable of learning your processes and rules or digest all of your training and knowledge articles in a few weeks will easily be able to adapt when they change. While testing might be more complex, this might change some of the design principles that underpin transformation projects going forward.
Some of the tools available now can generate personalised recommendations (for example, customer profiling and next-best-action in a sales environment). Martin talked about his experience of AI-powered training and coaching tools that learn their ‘client’ and provide genuinely personalised coaching advice.
- Take time to consider the people implications of what you are doing
Mark and Martin pointed out the risks of taking (or trying to take) the human ‘out of the loop’ when there are unclear business rules or important decisions that need to be made. Careful management of the potentially negative impacts of AI tools (i.e replacing human colleagues) is also vital for obvious reasons. Richard highlighted a different aspect of the potential people impacts where the use of automation and knowledge tools in a contact centre is having a positive impact on colleague satisfaction and performance as automated tools have absorbed some of the less interesting, repetitive work, leaving colleagues to handle more complex (more interesting) customer enquiries.
- Keep looking forward, be curious about what might be coming over the horizon
Two of our panellists mentioned things which weren’t possible (or affordable) five years ago but that are common now. The same is going to be true of AI and Automation over the next five years. Martin called-out personalised, augmented skills applications as something that is not far away. Other applications that were ‘pipedreams’ not long ago but are becoming more and more feasible include real-time (voice) translation and AI vision (search AI-powered shoplifter detection in store).
A non-technician can keep up to date by keeping close to a handful of the hundreds of on-line forums and experts sharing experiences (try the Global Business & AI Executive Forum and linkedin.com/in/pascalbornet for example).
Analysts like Gartner and consultancies like BCG publish regular market reviews, articles and case studies, which are often worth a read. Vendors and sales events are also useful, albeit that there is likely to be a bias in how they present features and benefits, with cross-business impact often underplayed.
We hope you find these helpful. Should you wish to find out more about any of these points, or you need independent guidance in identifying, selecting and implementing new technology, please don’t hesitate to get in touch.
Recent Posts